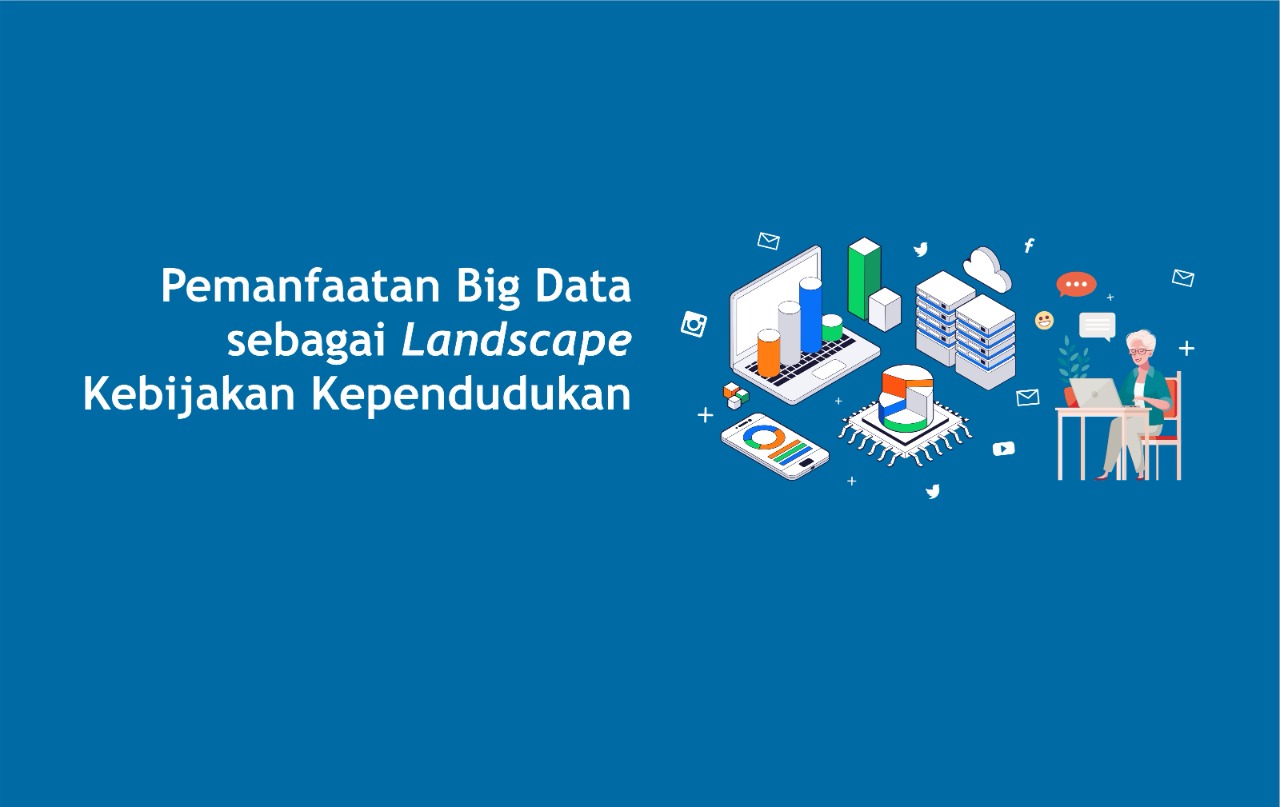
CPPS UGM – Population sector is often closely related to public policy terminology. Demeny (1988) defines population sector as a government activity with development planning that is understood and practiced as a centrally coordinated set of sectoral plans. The close relations between population and policy can also be seen from the policy concerning food, since population is also closely related to food availability. For this reason, the United Nations (UN) formed the United Nations Fund for Population Activities (UNFPA) (Bongaarts, 1994).
Both definitions show that the population sector in the public policy framework has been interpreted into institutional and system contexts. Population policy, which is often interpreted into both contexts, generates the discussion of population policy an empirical study, such as study of demographic characteristics, socio-economic conditions, transportation access, environment, and development (Alvarez-Diaz et al., 2018). In a more practical level, the discussion on population policy also includes ceremonial system and institutional activities.
In the context of Indonesia, for instance, population policy is seriously considered when the General Election (Pemilu) is held. The seriousness of data collection ahead of the general election is inseparable from the participation of the population in voting to make democracy a success, which is the interpreted into elections. Therefore, the issue of the Permanent Voter List (Daftar Pemilih Tetap/DPT) in the elections becomes a latent issue for the implementation of the General Election (Prayudi, 2018). Furthermore, the seriousness in thinking about population policy is also crucial for the government’s interest to update and maintain population data so that it does not fall into the hands of certain interests other than the context of the elections.
The seriousness is also found in health insurance policy. This is inseparable from the government’s obligation to provide basic social security for everyone and provide an adequate standard of living for everyone to obtain health and welfare services (Directorate of Protection and Community Welfare of Bappenas, 2014). The reach of everyone requires the government to collect data on population systematically and massively by placing the population not only by identity, but also by economic level.
Having provided with these examples, the government needs to collect data on population massively, quickly, and accurately. The data will be very important for the government to update data in the context of increasing DPT in elections, including providing social protection to the population. The need for population data quickly, precisely, and widely can be solved by using big data.
The use of big data in population policy is a new perspective in population policy. The perspective allows the government to monitor its citizens in real-time. A study conducted by Firdaus & Wijayanto (2020), for instance, shows that the use of big data, such as mobile-based population mobility, can be used to monitor individual compliance with health protocols during the Covid-19 pandemic. The study indicates that the use of big data is very useful to see how the effectiveness of other policy has been running, in this case is the Covid-19 health protocol. Thus, the use and development of big data is important to be developed within the population policy framework.
Broadly speaking, the author attempts to explore the use of big data in population policy by employing three things. First, the author aims to see the potential for using big data through social media and mobile phones as the development of Population policy. Second, the author highlights an example of a pension policy as a basis for explaining how big data is used. Third, the author emphasizes the importance of building digital demographics as the interpretation of big data products. The development of digital demographics is intended not only for the purpose of building live data, but also shows that multilinear data-based policy will produce comprehensive policy by taking the example of pension.
The Use of Big Data
Big data can be used as a basis for monitoring the implementation of policy. The use of big data is inseparable from several things. First, population events happen almost every day on a broad spectrum, both social events and economic events. These daily dynamics can no longer be responded to with manual population policy.
Second, almost every person in the world is connected to the internet and has their own mobile phone. Data from We Are Social (2021) shows that as of January 2021, there were around 4.66 billion people worldwide using the internet in January 2021 when the number increased by 316 million (7.3 percent) since last year. The data also indicates that in the same year, mobile phone users reached 5.22 billion people with a growth of 1.8 percent (93 million) since January 2020. Meanwhile, social media users also increased by 490 million over the past 12 months and reached 4.20 billion users.
The data indicate that there is great potential to observe population dynamics through monitoring the social activities of the population on social media and mobile phones. This step is possible to perform, given the internet, cell phones, and social media have links to each other. The three of them regularly make the users’ social activities update and will ask if the account owner is active for a few days or weeks.
Third, the big data development base can strengthen data-based policy. This is inseparable from the fact that big data can encapsulate various types of interrelated data into a policy cluster. Policy clusters are understood as a series of data that are related to each other, have a common pattern, and can be drawn a common thread within a geographical and organizational framework that is interconnected with related actors (The Innovation Policy Platform, 2010). It is important to establish situational mechanisms. When big data in the population sector can be developed later, the population mechanism is based on the population situation. To see the income differentiation based on gender, for instance, digital population data through social media in the form of online shopping expenditures, electronic banking data, and Population Identity Card (KTP) population data can be combined. Therefore, the policy can be comprehensive.
Having explained about the use of big data, there are several big data development opportunities. In this article, the author explains the potential of big data as the basis for developing pension policy and developing digital demographics.
Pension Policy
The development of big data is ultimately also important for human development. This is important because for developing countries, pension policy is often not in accordance with one’s dedication and performance. Therefore, a person is forced to continue to extend his/her career for the entire life. Whereas the extension of the career period may not be in accordance with his/her productivity. Such condition is in contrast to systems in developed countries where one’s rewards are clearly defined according to dedication and performance. Therefore, people have no worries while having their retirement.
These facts have opened a gap for big data to be able to build literacy and income security. The potential risk of income and expenses through a history of online shopping transactions, for instance, can be connected to the salary received by someone. This crossing data base will determine the amount of one’s take-home pay along with investment opportunities in the future when he/she retires.
Furthermore, big data can also be used to link the financial structure of pension policy with one’s level of education, including the level of one’s needs, the suitability of one’s skills with current work needs through LinkedIn site data, for example. Thus, people who are about to retire can be responded to with other investments, one of them is by working through digital platforms as freelancers or commonly called digital nomads.
Digital Demographic Development
The development of digital demographics is also one of the developments that can be achieved by using big data. This is inseparable from the urgency of big data development and data-based policy formulation. Furthermore, the development of digital demographics is not only needed to make data-based policy, but also comprehensive.
The development of digital demography is also needed to develop live data and formulate multilinear and comprehensive data-based policy. The development of digital demographics is similar to the social security system built in China. The social security system built in China has succeeded in reducing poverty when people who are not covered by public policy, and services in China can be identified through the current location of the population or satellite imagery for later intervention in poverty reduction policy by the Chinese government (Primayanti et al., 2017).
This utilization can be performed in Indonesia, although it is necessary to limit the private and public domains and their securities. The three require collaboration between actors in building digital demographics so that the private and public domains have clear boundaries, considering that the population problem is also a multi-sectoral problem.
This is important, considering that big data has a trend of data trafficking. Therefore, despite building collaboration to create a digital demographic platform that translates big data, segmentation or differentiation of public and private interests also needs to be considered.
Conclusion
Population policy cannot be made by manual methods and linearity approaches. The need to respond to population dynamics and build responsive data-based policy can be met through the use of big data. Big data is presented to provide comprehensive data on a problem, not just an issue. Problems tend to be multisectoral, so perspectives and data must also be multisectoral. Therefore, big data offers that.
Pension policy in Indonesia becomes a special lesson when retirement is not anticipated, such as how to transfer productivity and financial securities, only thinking about compensation which sometimes is far from one’s contribution, dedication, and performance. In the end, digital demography is built as the foundation of the future population policy platform. The policy does not look at the population in one dimension but is multidimensional which requires multidimensional responses that are also combined in perspectives and data in a data-based policy.
References
Alvarez-Diaz, M., D’Hombres, B., Ghisetti, C., Pontarollo, N., & Dijkstra, L. (2018). The Determinants of Population Growth and Density. In JRC Working Papers in Economics and Finance (Issue October). https://doi.org/10.4324/9781351291521-30.
Bongaarts, J. (1994). Population policy options in the developing world. In Science (Vol. 263, Issue 5148). https://doi.org/10.1126/science.8303293.
Demeny, P. (1988). Social science and population policy. Population & Development Review, 14(3), 451–479. https://doi.org/10.2307/1972198.
Direktorat Perlindungan dan Kesejahteraan Masyarakat Bappenas. (2014). Perlindungan Sosial di Indonesia: Tantangan dan Arah ke Depan. In Bappenas.
Firdaus, Z. F., & Wijayanto, A. W. (2020). Tinjauan Big Data Mobilitas Penduduk Pada Masa Social Distancing Dan New Normal Serta Keterkaitannya Dengan Jumlah Kasus Covid-19. Seminar Nasional Official Statistics, 1, 265–272. https://doi.org/10.34123/semnasoffstat.v2020i1.502.
Prayudi. (2018). Mengapa Masalah Dpt Terus Terjadi? In Info Singkat: Kajian Singkat Terhadap Isu Aktual dan Strategis: Vol. X (Issue 18).
Primayanti, L., Fasisaka, I., & Nugraha, A. (2017). Strategi Pemerintah Tiongkok Dalam Mengentaskan Kemiskinan Dan Kelaparan Yang Ekstrim Sebagai Target Pertama Mdgs. Jurnal Hubungan Internasional, 1(1).
The Innovation Policy Platform. (2010). Cluster Policy. https://doi.org/10.1007/978-3-030-69842-3_2.
We Are Social. (2021). Digital 2021: The Latest Insights into the “State of Digital.”
Https://Wearesocial.Com/Blog/2021/01/Digital-2021-the-Latest-Insights-into-the-State-of-Digital.
https://wearesocial.com/blog/2021/01/digital-2021-the-latest-insights-into-the-state-of-digital.
*Satria Aji Imawan, M.P.A. | Pengamat Kebijakan Publik UGM, Dosen dan Staf Akademik di Magister Kepemimpinan dan Inovasi Kebijakan UGM | Ilustrasi: M. Affen Irhandi/PSKK UGM | Editor Bahasa: Rinta Alvionita
*Tulisan ini merupakan refleksi dari pemaparan hasil penelitian terkait Anticipating the Future pada APA Conference 2021